Domain-Specific vs. General LLMs: Why Beehive AI Outperforms ChatGPT in Analyzing Customer Data
- Beehive AI
- Aug 27, 2024
- 5 min read
Updated: Dec 17, 2024
TL;DR
The advantages of using a specialized model like Beehive AI for analyzing customer data as opposed to a general-purpose LLM, like ChatGPT, include:
Privacy and security: a private, secure LLM instance where your data is only used to train your model
Higher accuracy, relevance, consistency, and reliability: fine-tuned model that is fact checked by humans and is grounded in your data means more accurate, relevant, consistent, and reliable answers
Enhanced granularity and nuance of the results: you get more information from the data analysis
Accurate mathematical capabilities: combined statistical analysis alongside generative AI capabilities(e.g., quantifying issues)
Test it for yourself. Sign up to try Beehive AI for free!
Full Story:
In our initial meetings, customers often ask us about the difference between analyzing customer data on our platform versus using a general-purpose LLM like ChatGPT. As you can imagine, we’ve done our testing and we share that info with customers in those meetings. We are sharing our experience to help people and, hopefully, save them time when they are evaluating options.
Generative AI has been the hottest technology since it arrived in 2022. And people find the public large language models incredibly useful - I’m using one right now to help write this article. However, like anything in life, no single solution solves all problems. As the saying goes, "When you have a hammer, everything looks like a nail." In the case of analyzing a company’s customer data, general purpose, public LLMs are not the best solution.
To illustrate, we used a public dataset of mobile app reviews for a leading car manufacturer. We removed references to the brand in the illustrations. We encourage you to run your own tests. You can sign up to Beehive AI here.
Even before considering the quality of the analysis, one major concern with public LLMs is the uncertainty around data handling, data security, and privacy. Most companies prohibit uploading customer data into a public LLM. And, even for enterprise versions of the public LLM, CTOs have many questions about how the LLM is handling their data. We’ve received the same questions.
In contrast, Beehive AI isolates your data in a private, secure environment and only uses your data to train your model. Unlike general public LLMs, your data does not go back into the Beehive AI LLM. And Beehive AI gives you the option to delete your data at any time.
Ok, onto the results from our tests.
The first issue we experienced with the general LLM was inaccuracy. While much attention is given to hallucinations, LLMs also make 'honest' mistakes. For example, we were surprised when ChatGPT suggested that an app drained the car battery. This did not make sense. And we could not investigate the issue through more prompting - ChatGPT kept giving the same response.
We did the same investigation on Beehive AI and we found that it was actually the mobile app draining the phone battery, not the car battery.
This is an example where the limitations of general-purpose LLMs are more apparent. In this case, the general-purpose LLM was confused and provided an inaccurate response, mainly because it is built on a probabilistic model using a much larger dataset, unlike platforms like Beehive AI, which are built on smaller, industry or customer specific datasets.
To provide more details, accuracy is achieved with Beehive AI through a few mechanisms:
Specialization, i.e. fine-tuned model specifically for analyzing customer data
Context, i.e. a specific adapter each company gets fine-tuned for the company data (and domain)
HITL, i.e. fact-checkers statistically verifying the categorization of data
Exhibit 1: Visuals from our investigation into what was draining the battery.
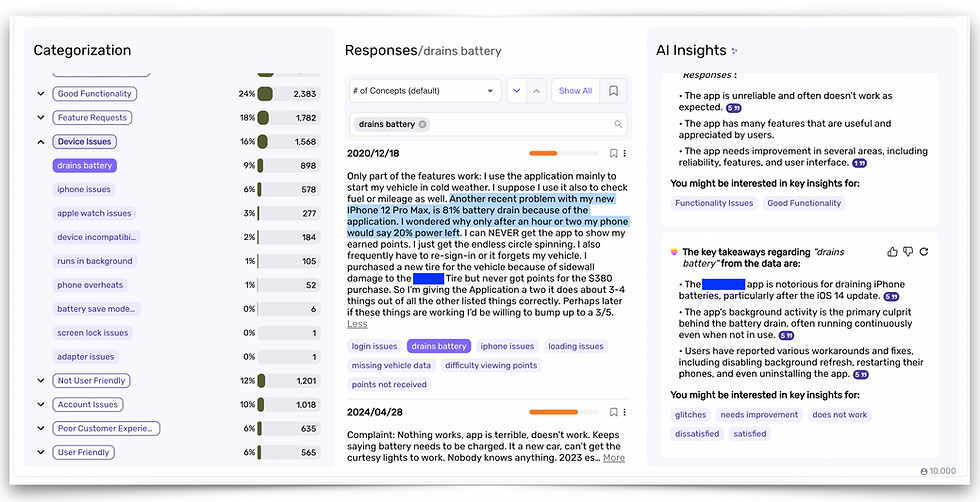
Exhibit 2: ChatGPT incorrectly thinks that the car battery is being drained.
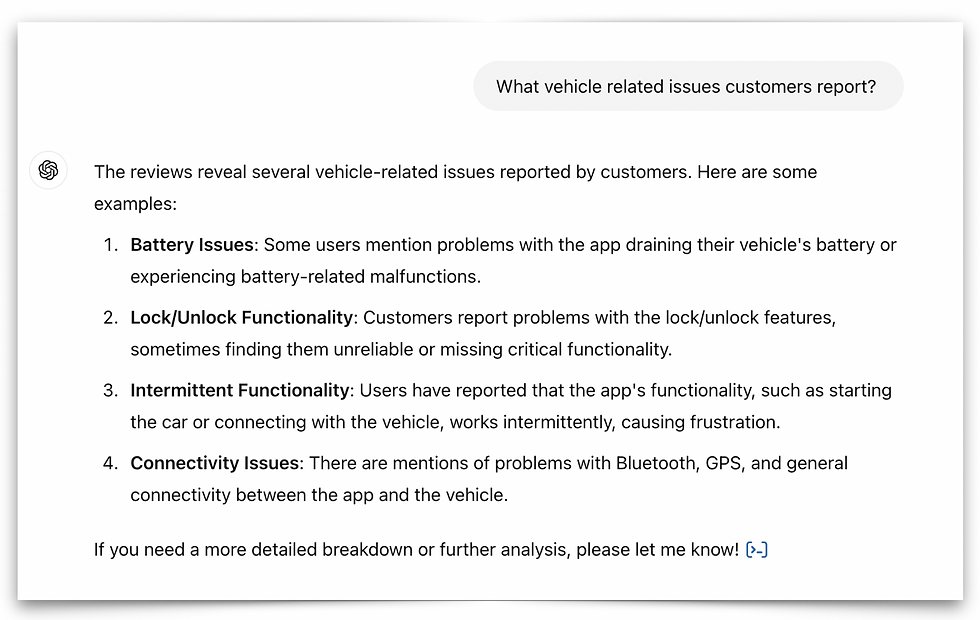
The second issue we noticed was in terms of consistency. When we uploaded a data file to ChatGPT and asked the same or similar questions multiple times, we got different responses. This may be because general purpose LLMs incorporate a degree of randomness to make responses more varied and more human-like. This may also be happening because of the way the LLM was trained (e.g., temperature setting). Regardless of the reasons, when you are trying to make strategy, product, or marketing decisions you want consistency in the analysis and ChatGPT did not return consistent responses.
Exhibit 3: Asking about vehicle-related issues customer report twice gave us two different set of issues.
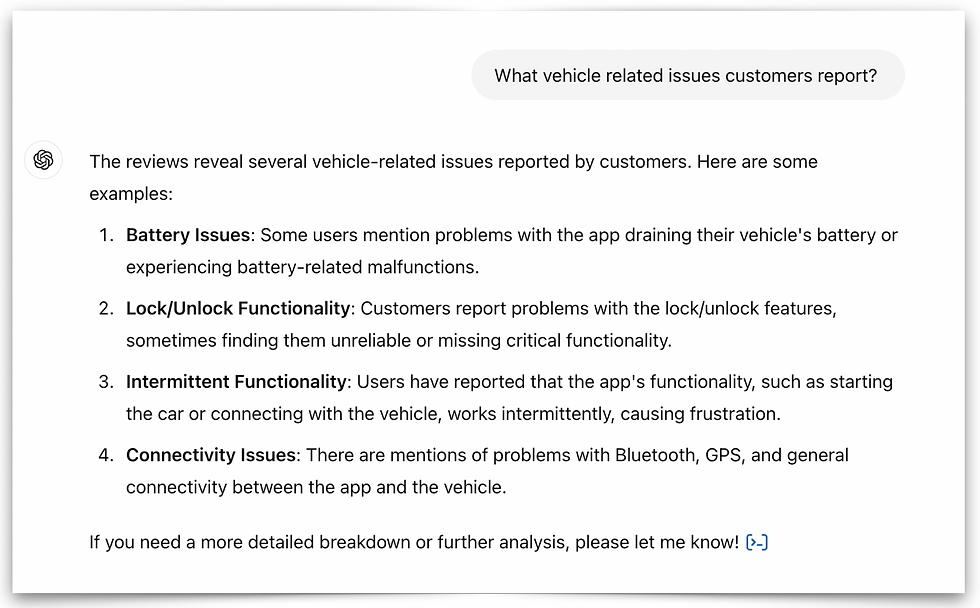
Exhibit 4: And the responses after the second time we asked the same question.
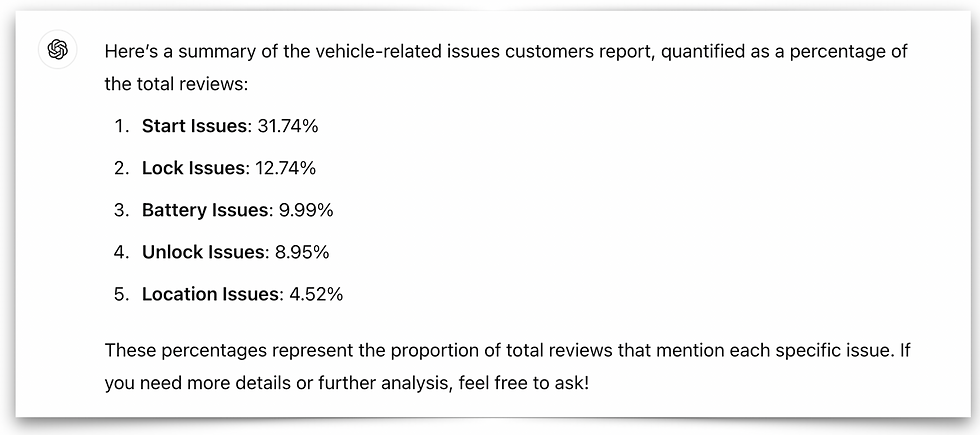
On the other hand, with Beehive AI, the results were consistent. The reason for this is that with Beehive AI, consistency is enforced through supervised fine-tuning and grounding an adapter (bespoke) model to a verified structured index.
The third issue we experienced was granularity. General models are good at capturing big, general trends; however, it takes a specialized model to capture the nuanced complexity of the data and trends.
In the examples below, you can see the vast difference in richness between the detailed categorization of functionality issues that Beehive AI produced from the data and the generic, coarse list created by the general-purpose ChatGPT.
The most important finding about issues, ‘problems with the app after updates,’ doesn’t even appear in ChatGPT’s analysis, and even smaller issues (3%), like ‘notification issues,’ are missing. The granularity of the Beehive AI model comes from its specialization, fine-tuning, and rigorous fact-checking process.
Exhibit 5: We asked ChatGPT to find functionality issues that customers reported the most.
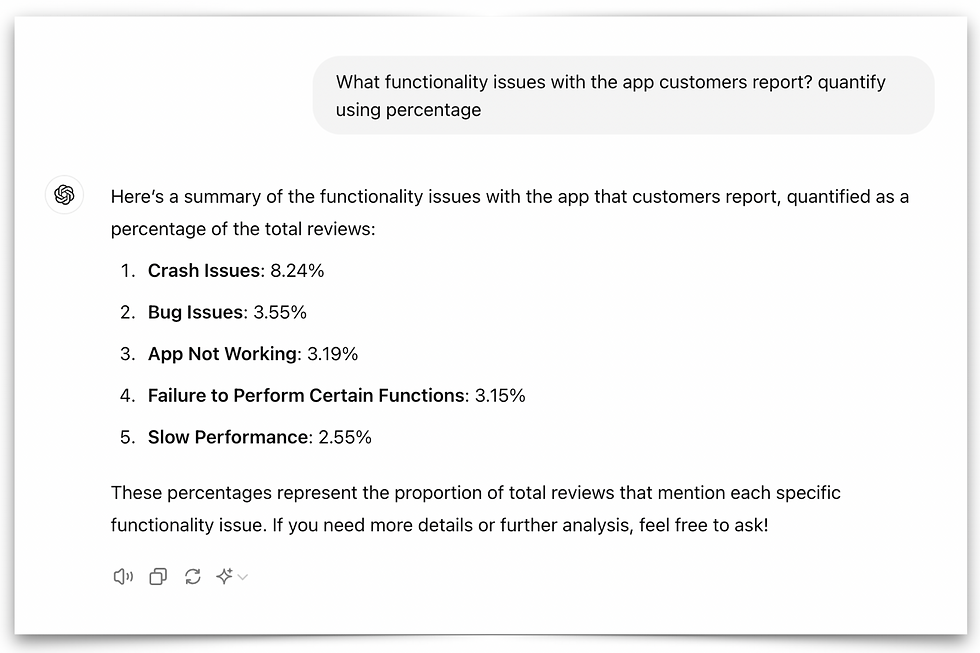
Exhibit 6: Beehive AI finds functionality issues that customers reported the most.
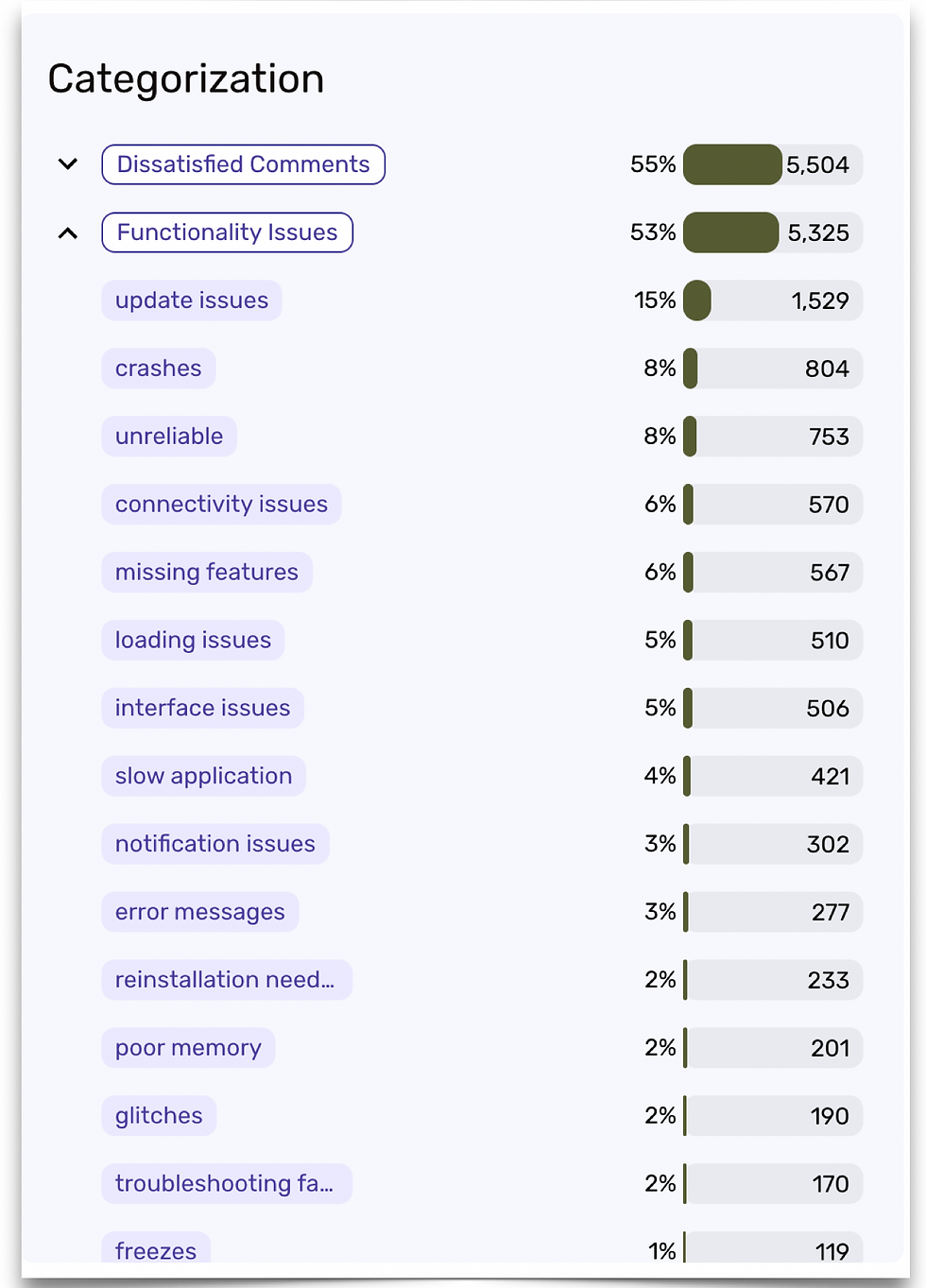
The last issue worth mentioning was about quantification and segmentation/comparison. It’s one thing to list issues and opportunities, but it’s another to quantify them accurately so you can prioritize and understand the magnitude of the problem or opportunity. LLMs do not do math well; a quick search will return articles explaining why.
Beehive AI is a full statistical analytical platform with statistical significance and has robust features you would expect from a statistical tool. Furthermore, it relies on verified and tested categorization to ensure accurate counts and ground the LLM. Asking a general LLM to do math, segment, compare, and quantify differences means you are just as likely to get the wrong answer as the right one. At Beehive AI, we base these operations on proven statistical models to ensure accuracy. And we combined that with our fine-tuned generative AI LLM.
In conclusion, when you are looking to make accurate business, strategy, product, marketing, sales, decisions, you are better off using a model that was trained and built for purpose than relying on a general, public model. The key elements to consider are: 1) privacy and security: how is my data being handled, 2) accuracy, consistency, and reliability: how is the LLM built and how is it analyzing my data, 3) granularity and nuance: is the model returning more and deeper information, and 4) model sophistication: is the model combining statistical and generative AI capabilities. Evaluating these factors will help you find the right AI model for your organization.
Comments